Brain-Computer Interface is a technology which is letting the human brain and the external devices communicate with each other to take the respective actions intelligently. It is a kind of brain neural controlling interface and is often called BCI. This technology is widely helping paralyzed individuals as this is in no need of physical movements. In addition, they never hinge on the exterior nerves/muscles & common output pathways. “This is the article which is proposed to bring the significant facets of the brain computer interface project ideas”
Various technologies have been proposed to connect the digital and the physical world environment. Besides, some of the technologies only attained the connectivity between the two dominions in which brain computer interface is one of the major irreplaceable technology that is reading the human mind as well as converts the neuron signals in the form of desired output as per users’ requisites.
EEG is the electroencephalogram signal which is the best choice for BCI. At the end of this article, you will be educated in the various fields that are essential for conducting the projects in BCI. We are promising that you are going to enjoy this article very much. Now, let’s light up this article with the outline of the brain computer interface.
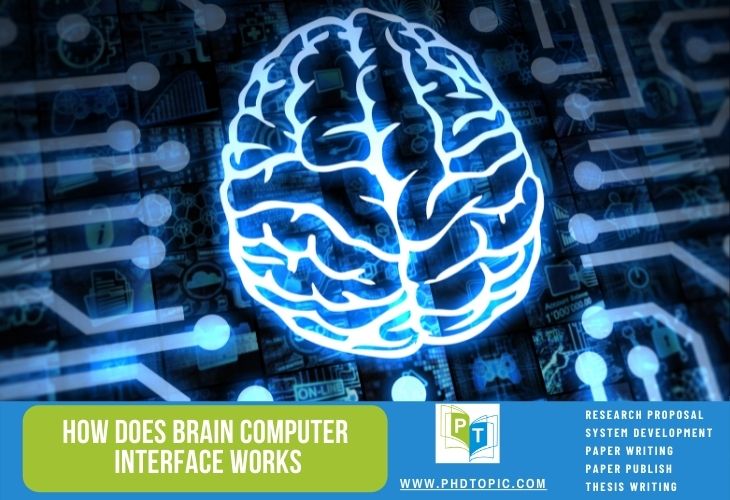
Outline of Brain Computer Interface
BCI is completely a thought-oriented technology that controls external devices. The devices can be any computerized peripherals such as cars, electronic equipment, etc. Brain signals are captured and broadcast to the external devices by the electrodes. Brain signals are converted into data then translated to the devices which are ported.
This is the crisp overview of the brain computer interface project ideas. This article is concentrated on simplicity with high-quality stuff to reach every student from all over the world. Primarily, we have seen about the overview then we can know how does a brain computer interface work to make your understanding better.
How Does a Brain Computer Interface Work?
Brain signals are the core component which is interpreting the BCI records. In addition, an electrical signal that is produced in the brain is transmitted over the neurons for communication. The central nervous system gets the signals from the brain to pass out the actions that are to be performed by the muscles. Brain signals can be captured in some of the ways,
- EEG Signal Acquisition & Processing
- EEG amplifiers/speakers are permitted to access the archived signals
- Electro-Sensors
- Sensors are the electrodes embedded over the scalps of the skull
- Communication Translation
- It can be done by significant commands & script languages
This is how being signals we hustled in general. Our technicians in the crew are very accustomed to these areas hence the illustrating capacity of our experts is incredible. We thought that it would not be enough to make you understand since we have tried our best to stimulate your intelligence by our demonstration of how a BCI does exactly works. Come on dear students, lets we try to understand them to step by step.
- Step 1: Acquisition of Signal
- EMG based Signals (Muscle Actions)
- EOG based Signals (Eye Actions)
- EEG based Signals (Electrical)
- fNIRS, HbR & HbO based Signals (Optical)
- Step 2: Feature Extraction
- EEG based Features (PSD & Peak)
- fNIRS based Features (Slope & Average)
- Hybrid / Combined Features (HbR & HbO)
- Step 3: Classification
- EEG based Classifiers (Control Commands)
- fNIRS based Classifiers (Control Commands)
- Hybrid / Combined Classifiers (Accuracy)
Eye movement-based EOG signals are used to identify the eye actions and the noises in the blinks are removed by the feature extraction phases. In addition, eye actions or movement-based commands are widely used in the EOG classification areas. On the other hand, muscle movements are handled by the EMG signals and the noise was removed from the feature extraction processes. Classification of the BCI system genuinely increases the possibilities to raise the control commands as well as inaccuracy. Moreover, the BCI system is categorized under 3 types as listed in the below section.
Types of Brain Computer Interface
- Passive BCI Systems
- Active BCI Systems
- Reactive BCI Systems
The aforementioned are the 3 types of brain computer interface systems. At this time, we felt that it would be highly beneficial to the beginners in this technology by stating how to capture the brain signals. The aforementioned are some of the brain computer interface project ideas, apart from this there are so many deep-seated ideas that are drifting in our hands. Yes, you people guessed right!!! We are going to let you know the same with clear points.
How to Capture the Brain Signals?
Brain signals can be captured by the application of neuroimaging tools and some of them are listed with the essential features and descriptions.
- fNIRS
- fNIRS processes the subjects in a very slow manner
- It is effortlessly portable with other external devices
- It has a high spatial resolution & temporal resolution of up to 1s
- MEG
- MEG scanners do not have any computer devices to visualize
- It is not compatible with the portability to other devices
- It is also havinga high spatial resolution & temporal resolution of 0.05s
- FMRI
- FMRI based scanners are motionless & reveal the high artifact images
- Portability with the other devices is likely makes disappointments
- The spatial resolution of this tool is always high
- The temporal resolution of the FMRI tool is overlaying up to 1s
- PET
- PET tools analyse the perilous properties of the scanning
- Connectivity with the other external devices is a strict no
- Here spatial resolution is high & temporal resolution is 1s
- EEG
- EEG scanning makes use of the gels & needs motionless features
- Computerized devices such as external types of equipment will interfere
- It can connect with other devices
- It has a low spatial resolution & temporal resolution like 0.05s
Brain signals are being processed & measured by several tools such as mentioned in the following arguments. There are 3 ways in which we can process the electrical signals that are emanated by the human brain. In the upcoming section, we have illustrated how to process the signals in BCI for the ease of your understanding. Come on dears, let us try to understand them.
How to Process the Signals in BCI?
- Invasive
- The implantation of electrodes in the cortex offers intraparenchymal signals
- Non-invasive
- Placing electrodes on top of the scalp effectively expose the EEG signals
- Semi-invasive
- Embedding electrodes in the areas of arachnoid/dura can offer the ECoG signals
These are some of the significant ways in which BCI-based communication can be done. These processes are very essential when conducting BCI-based researches. At this time, we would like to furthermore state the steps involved in the above-listed areas particularly about the EEG in BCI standards.
- Acquiring Signals
- Noise Removal
- Segmentation of Bands
- Extracting Features
- Classification
This is how the brain signals are processed principally in EEG. Further, we hope that you are getting the points as of now enumerated. However, every technology in the technical world is pampered with some pros and cons likely; a BCI system does have some of the challenging issues while processing the brain signals to the external devices as determined output. Let’s have further issues of the BCI in the next section.
What are the Challenging Issues of BCI?
- Lack of artifacts presence from exact sources
- Inefficient electronic noises with voltage barricades
- Low frequent & modifications in the signal of interest
- Variations & fluctuations in biological concentrations
- Disorganized responses from electrodes to energy modalities
In the summary, the noises/artifacts interferences to the BCI are presented from various unfamiliar peripheral and other internal sources of the human bodies. Shot noise & thermal noise are the two major classes of electronic noise which rely on the semiconductor’s voltage barriers. On the other hand, the interest of the signal is determined by the aspects such as tongue actions, vibrations, skin textures, and mainly breathing.
Moreover, electrophysiological fluctuations like EMG, ECG & EOG are also bothering the physiological variations. Finally, the artifacts in the transducer arise when electrodes retort to the energy modules. As this article is titled with the brain computer interface ideas, we wanted to cover up the next section with the same for your references.
Latest Brain Computer Interface Project Ideas
- BCI using Deep Learning Concepts
- Neural Networks based System Specific Understanding
- fNIRS& EEG Multimodal based BCI
- Brain Signal Classification using Machine Learning
- Brain Signal based Emotion Classification
If you are looking for dynamic project assistance with innovative perceptions then this is the best platform ever. At this time, we would like to talk about the deep learning technologies’ contribution to the brain computer interface technology for the ease of your understanding.
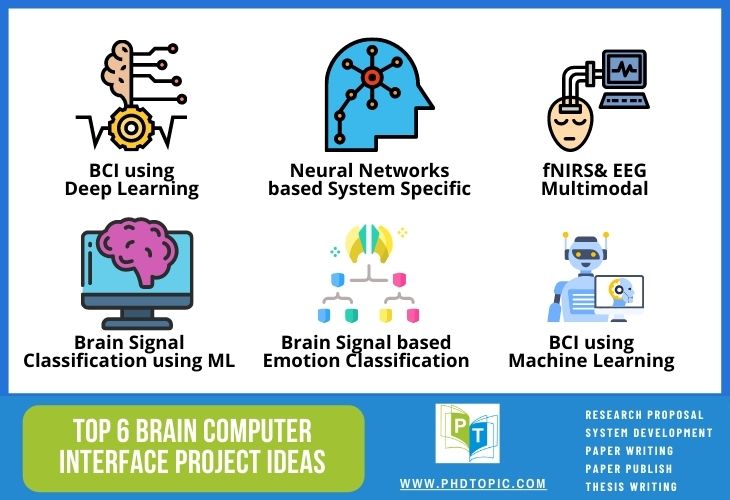
The signals emitted from the human brain are subject to physiological exploitations. These could be caused by the level of concentration, mental illness; muscle noises & eye blinks even from environmental noises. Electroencephalogram is recently failed to face the constraints that arise during the signal acquisition whereas deep learning-based techniques and algorithms are widely considered as resilient for brain signal identification. In this regard, let us discuss the deep learning application in BCI systems in clear points.
Deep Learning for Brain Computer Interface
- Deep learning techniques are replacing the application of LDA & SVM classifiers
- The backpropagation technique is used to segregate the brain signals into valid info
- Deep learning is effectively preprocessing & extracting the brain features
- It is highly capable of recognizing the hidden & high resolute features
In crisp, deep learning is one of the extremely rare technologies which is uniquely pinpointing the challenges that arise in the BCI systems. BCI systems are widely facing classification issues that are subject to high accuracy levels.
Hence, the traditional classifying algorithms such as SVM (Support Vector Machine) & LDA (Linear Discriminant Analysis) are failing to classify the raw brain signals. In the end, we are emphasizing deep learning technology as the optimum selection for BCI systems. In this regard, let us also have a quick insight into some of the deep learning algorithms that are used to the brain computer interface.
Deep Learning Algorithms for BCI
- Recurrent Neural Networks
- Classification & Feature Learning based Hybrid Models
- Deep Belief Networks
- Generative Adversarial Networks
- Variational Auto Encoder
- Convolutional Neural Networks
The itemized above are the diverse deep learning-based algorithms for the brain computer interface systems. At this time, bringing back the short overview of these algorithms would make your understanding better. Yes, dears we are going to let you know about the further explanations of some algorithms for the ease of your understanding.
- Convolutional & recurrent neural networks are highly compatible with the spatial & temporal “feature extraction”
- Deep belief networks are capable of handling the feature extraction even if there is a “dynamic feature” espousing in hybrid & CNN models
- “Augmentation of data & training samples” can be engendered by generative adversarial networks
- CNN has the “scalability” feature and which can showcase the hidden spatial based addictions from EEG & fMRI scanned brain images
In short, training data quality can be enriched by the application of generative adversarial networks. Moreover, brain signal batches are renovated by adversarial networks. These are some of the explanations of the above-listed deep learning algorithms. If you still want any clarifications to these extents you could approach our researchers at any time, we are here to help you out. Following these phases, we would like to educate you in the areas of tools that are used in the BCI systems for the ease of your understanding.
Tools and Software for Brain Computer Interface Projects
- BCILAB- Matlab
- PyFF- Python
- PsychToolbox- Matlab
- OpenVIBE- C++
- BCI2000- C++
- BciPy- Python
- PsychoPy- Python
The above listed are some of the widely used toolkits for brain computer interfaces projects. This section will be very important as selecting the brain computer project ideas because it will be helped to implement the determined aspects. These tools are significantly compatible with the python bindings & primary BCI modules. On the other hand, some of them also are not supported with the BCI modules which are primary, and mainly PyFF has not tribute anything in the last years as its contribution.
Generally, embedded systems are mostly using python since they can design the compound techniques. Python is one of the user-friendly languages which has simplified syntaxes as well as bridges the connectivity between the machine learning libraries. In this regard, let us see such ML libraries for the ease of your understanding.
- Hcluster
- Modular Data Processing
- PyML
- Orange
- Shogun
- Elephant
In short, agglomerative clustering is dealt with by the Cluster ML libraries whereas PyML is the library that has the features of machine learning in python. In addition, data mining techniques are widely dealing with the Orange machine learning libraries in general while Shogun & Elephant toolboxes are complete and multi-purpose machine learning toolboxes correspondingly.
So far, we have discussed the necessary facets that are essentially presented while handpicking brain computer interface project ideas. Doing researches and projects in these areas would bring you the best results beyond your determined extend. For this, you can approach skilled experts or technicians in the industry because they know the constraints that arise during the phases of research. If you are interested you can also prefer our researchers at any time for paper writing service.
“Let’s make use of every opportunity to showcase your capabilities in the areas of technology”